Your computer is watching you, but chances are if you’re a person of color, researchers say it could also be making up a stereotypical storyline to accompany the view.
Every major technology company uses machine learning models to perform better. From the suggestions your shopping app makes while you search for your next pair of jeans to the movies your streaming service says you should watch next, all of the platforms we rely on daily are fueled by recommendation algorithms. A programmer “teaches” the machine by feeding it data. Every time you confirm you are a human and not a robot while doing business online, you’re helping to train the artificial intelligence. However, according to experts, these tools are making decisions based on fundamentally biased data, which can adversely affect a person’s civil rights and even lower the number of opportunities presented.
“Data plays a very big role in artificial intelligence and machine learning,” says Danda Rawat, PhD, Howard University engineering associate dean for research, professor, and director of Howard’s Data Science and Cybersecurity Center.
The 2018 groundbreaking paper, Gender Shades: Intersectional Accuracy Disparities in Commercial Gender Classification, detailed the rampant bias currently displayed by machine learning. Researchers Joy Buolamwini from MIT and Timnit Gebru from Microsoft examined a data set of 1,000 images of faces of those from three African countries and three European countries, putting IBM, Microsoft, and Face++ to the test. Each performed its worst on darker-skinned females, meaning the programs repeatedly could not “understand” or see the images of women or men of color, while it could quickly decipher those of white men and women.
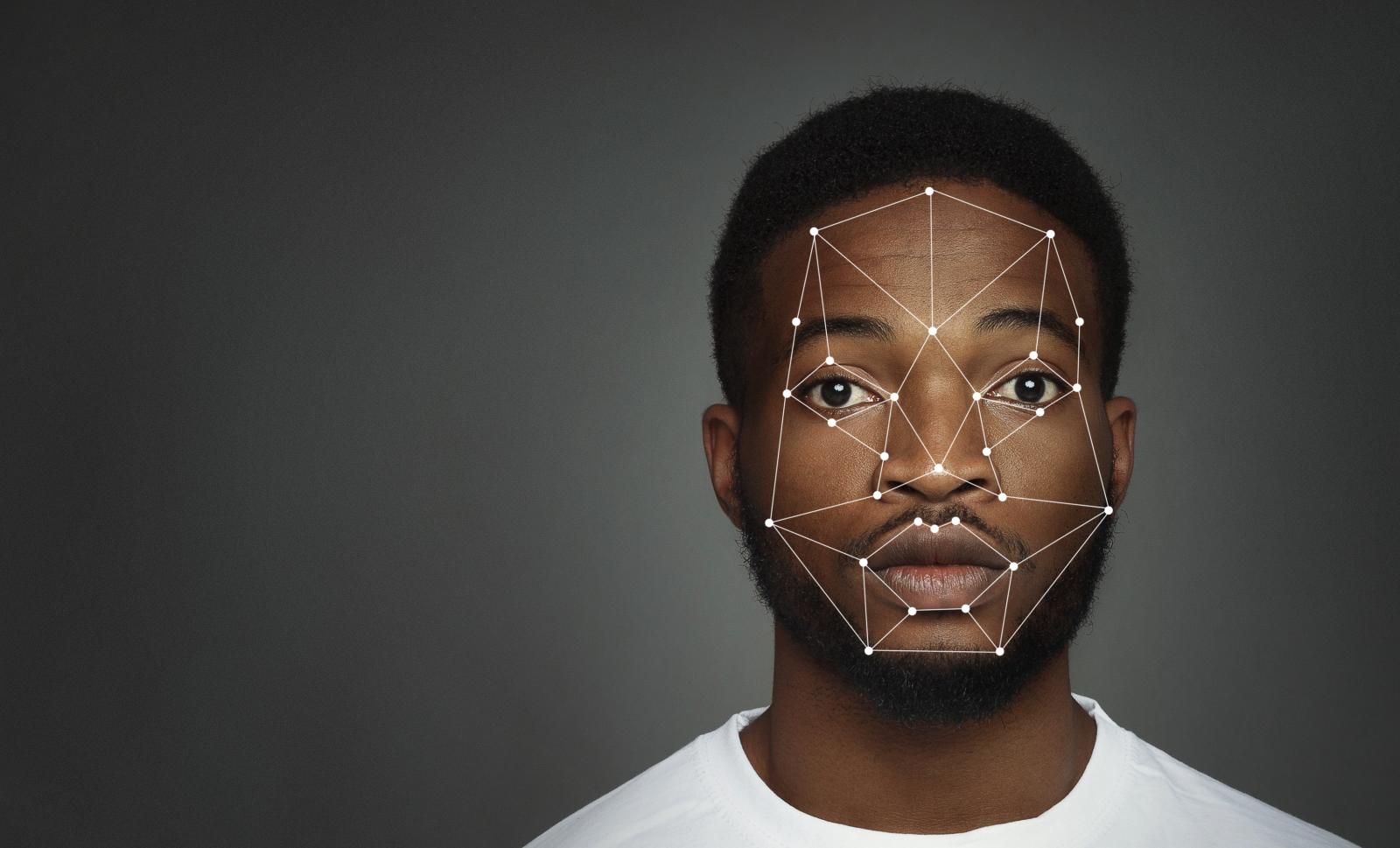
It’s an action born from the lack of diversity in data used to train the machines and could translate into bias, affecting who is hired, who is accused, and who receives access to quality medical care. For example, researchers say if the machine reviews a name or face and assigns a negative connotation to that person or resume, it could cause a series of negative reactions, affecting that particular person who doesn’t get the job and now can’t afford to feed his or her family.
Artificial Intelligence is Not Intelligent
“When you say AI, artificial intelligence, you’re implying that this construct has intelligence, you’re assigning a human quality to this machine and these machines are not human,” cautions Dhanaraj Thakur, PhD, an instructor in the Department of Communication, Culture and Media Studies at Howard University. “They can’t empathize. They can’t deal with ethical issues or moral issues, so that implies questions around justice and fairness.”
Thakur is also research director at the Center for Democracy and Technology (CDT). His work has examined automated content moderation, data privacy, and gendered disinformation, among other tech policy issues. Thakur says research has shown that machine learning tools can be highly discriminatory and biased towards people of color in particular. In one example, different facial recognition tools were shown to be less accurate when it came to classifying darker skinned women compared to other groups. In another example, when asked to complete sentences about Muslims, a machine learning model returned results that were often violent and linked to terrorism.
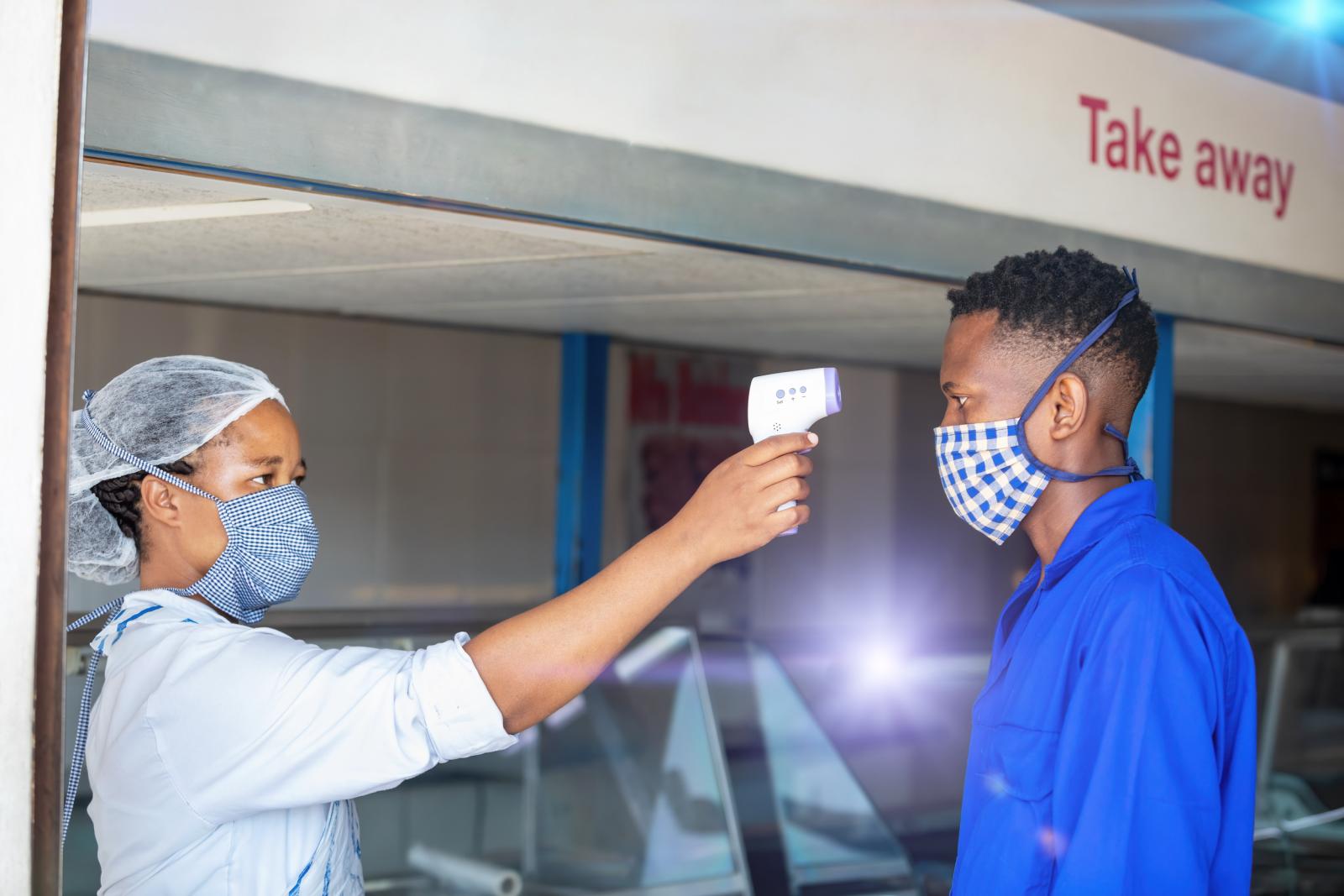
“Let’s say it’s not intentional. Why is it happening?” questions Thakur. “Three reasons: these models are often developed by a like-minded group of programmers, usually white men. When you talk about machine learning developers, they are not diverse in the way the U.S. is diverse. Second is the data sources and the data quality. These datasets include millions and billions of data points. They’re huge, yet they’re often still not representative of society in many ways. And then finally, most of the content on the web – and so most of the data used to train these models – is in English.”
They can’t empathize. They can’t deal with ethical issues or moral issues, so that implies questions around justice and fairness.”
His third point is the impetus for the current research focus of his research team – the use of large language models in non-English languages. As the team noted in a recent blog post: “Large language models are models trained on billions of words to try to predict what word will likely come next given a sequence of words (e.g., “After I exercise, I drink ____” → [(“water,” 74%), (“gatorade,” 22%), (“beer,” 0.6%)]).” The team found that when it comes to the use of these models (like ChatGPT) the relative lack of data, software tools, and academic research in non-English languages can lead to serious problems for some populations. For instance, advocates have argued that Facebook’s failure to detect and remove inflammatory posts in Burmese, Amharic, and Assamese have promoted genocide and hatred against persecuted groups in Myanmar, Ethiopia, and India.
Instead of collecting new data that is wholly representative of society, the machine selects the answer that best fits the data set, but what if the selected collection of data is wrong or not large enough to accurately represent the world?
Combatting racism in AI
Researchers at Howard are on the frontlines of fighting racism displayed in the artificial intelligence that big tech companies use and share.
Howard made history earlier this year, announcing it will lead one of the Air Force’s 15 university-affiliated research centers, the first HBCU to do so. The five-year project includes forming a consortium of historically Black colleges with engineering and technology capabilities.
A UARC is a United States Department of Defense research center associated with a university. The cutting-edge institutions conduct basic, applied, and technology demonstration research. The partnership allows for a rich sharing of expertise and cost-effective resources. Rawat serves as the executive director of the UARC and principal investigator on the Howard contract.
“If we don’t trust the system, it’s not usable by the people,” says Rawat. “We have to change that.”
Article ID: 1371